Hyperspectral and Multispectral Imaging
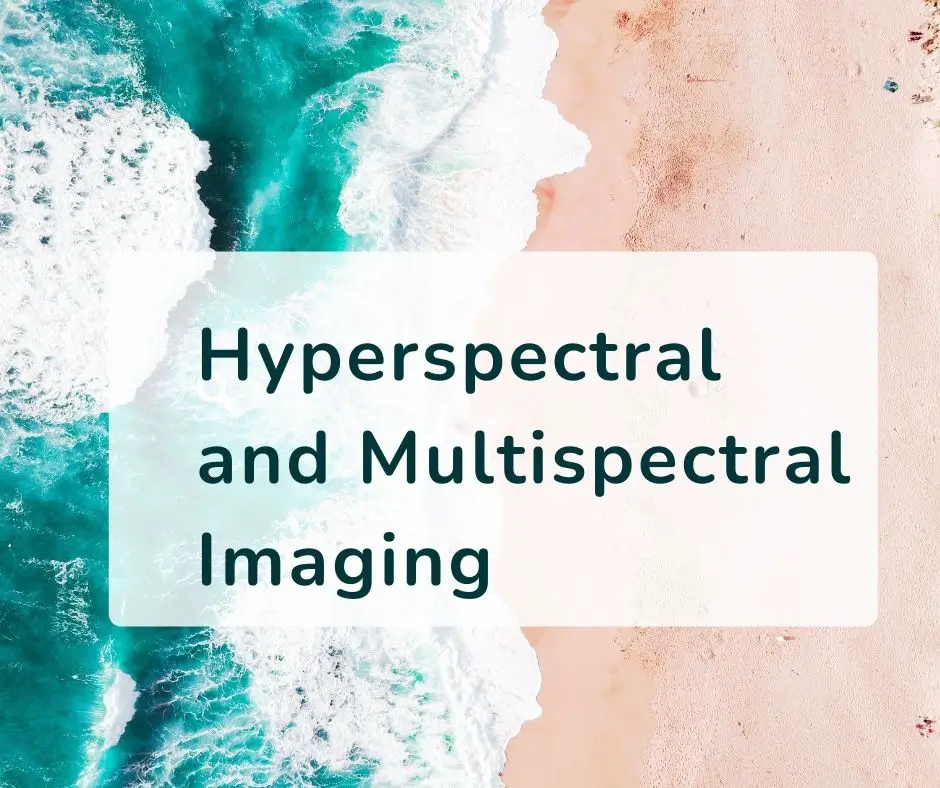
Hyperspectral and Multispectral Imaging
Hyperspectral Imaging
Hyperspectral imaging, like other types of spectral imaging, captures and processes data from the whole electromagnetic spectrum.
The goal of hyperspectral imaging is to collect the spectrum for each pixel in a scene image in order to find objects, identify materials, or detect processes.
Spectral imagers are classified into three types. Push broom scanners and related whisk broom scanners (spatial scanning) read images over time, band sequential scanners (spectral scanning) capture photos of an area at multiple wavelengths, and snapshot hyperspectral imaging generates an image in a moment using a staring array.
Whereas the human eye perceives visible light color in three bands (long wavelengths as red, medium wavelengths as green, and short wavelengths as blue), spectral imaging divides the spectrum into many more bands.
This approach of separating photos into bands can be applied to images that are not visible. The recorded spectra in hyperspectral imaging have precise wavelength resolution and encompass a wide range of wavelengths.
In contrast to multiband imaging, which measures dispersed spectral bands, hyperspectral imaging captures continuous spectral bands.
Hyperspectral imaging Sensors
In a figurative sense, hyperspectral sensors collect data as a series of ‘pictures.’ Each image represents a spectral band, which is a limited wavelength range of the electromagnetic spectrum.
These ‘images’ are combined to generate a three-dimensional (x, y,) hyperspectral data cube for processing and analysis, where x and y represent the scene’s two spatial dimensions and represents the spectral dimension (comprising a range of wavelengths).
Sensors can sample the hyperspectral cube in four ways: spatial scanning, spectral scanning, snapshot imaging, and spatio-spectral scanning.
Methods Of Scanning In Hyperspectral Imaging
There are four fundamental approaches for getting the three-dimensional (x, y, ) dataset of a hyperspectral cube.
The technique chosen is determined by the specific application, as each technique has context-dependent advantages and disadvantages.
Spatial Scanning
Each two-dimensional (2-D) sensor output in spatial scanning represents a whole slit spectrum (x,).
Slit spectra are obtained by projecting a strip of the scene onto a slit and dispersing the slit image with a prism or grating in hyperspectral imaging (HSI) devices for spatial scanning.
The disadvantage of these systems is that the picture is processed per line (through a push broom scanner) and that some mechanical pieces are included into the optical train.
Spectral scanning
In spectral scanning, each 2-D sensor output represents a monochromatic (‘single-colored’), spatial (x, y) map of the scene.
HSI devices for spectral scanning are typically based on optical band-pass filters (either tunable or fixed).
The scene is spectrally scanned by replacing one filter after another while the platform remains motionless. If there is movement in the scene, spectral smearing can occur in such ‘staring’ wavelength scanning devices, invalidating spectral correlation/detection.
Nonetheless, there is the benefit of being able to select spectral bands and having a direct representation of the scene’s two spatial dimensions.
Non-scanning
A single 2-D sensor output contains all spatial (x, y) and spectral () data in non-scanning. Non-scanning HSI devices produce the entire data cube at once, with no scanning.
A single snapshot, in figurative terms, represents a perspective projection of the data cube, from which its three-dimensional structure can be reassembled.
The snapshot advantage (greater light throughput) and quicker acquisition time are the most noticeable advantages of these snapshot hyperspectral imaging systems.
Spatiospectral scanning
Each 2-D sensor output in spatiospectral scanning represents a wavelength-coded (‘rainbow-colored,’ = (y)), spatial (x, y) representation of the scene.
A camera at some non-zero distance behind a simple slit spectroscope (slit + dispersive element) is used as a prototype for this technology, which was introduced in 2014.
By adding a dispersive element in front of a spatial scanning system, advanced spatiospectral scanning systems can be obtained. Scanning can be accomplished by moving the entire system relative to the scene, the camera alone, or the slit alone.
Spatiospectral scanning combines some of the advantages of spatial and spectral scanning, while mitigating some of their drawbacks.
Multispectral imaging
Multispectral imaging gathers visual data across the electromagnetic spectrum at certain wavelength ranges.
The wavelengths can be separated using filters or detected using devices sensitive to certain wavelengths, such as light from frequencies outside the visible light range, such as infrared and ultra-violet.
Spectral imaging can extract information that the human eye cannot catch with its visible sensors for red, green, and blue.
It was designed originally for military target identification and reconnaissance. Early space-based imaging systems used multispectral imaging technologies to map Earth’s details such as coastal limits, vegetation, and landforms.
Document and artwork analysis have also benefited from multispectral imaging.
Multispectral imaging detects light in a small number of spectral bands (usually 3 to 15). Hyperspectral imaging is a subset of spectral imaging in which hundreds of continuous spectrum bands are often available.
Classification: Multispectral imaging
In contrast to other aerial photographic and satellite image interpretation projects, these multispectral images do not allow for straightforward identification of feature type by visual inspection.
As a result, remote sensing data must first be categorized, followed by processing using various data enhancement techniques to assist the viewer in understanding the information included in the image.
Depending on the classification algorithm utilized, such classification is a complicated task that requires rigorous validation of the training data. There are primarily two types of approaches.
- Supervised classification techniques- Training samples are used. Training samples are regions on the ground where there is ground truth, that is, what is known.
The spectral signatures of the training areas are utilized to look for comparable signatures in the image’s remaining pixels, and we classify accordingly. This method of using training samples for classification is known as supervised classification.
- Unsupervised classification techniques- In the case of unsupervised classification, no prior knowledge is necessary to classify the image’s features. The natural clustering or grouping of pixel values, i.e., pixel gray levels, is noticed.
The number of classes in the image is then defined as a threshold. The more classifications there are, the finer the threshold value.
Beyond a certain point, however, the same class will be represented in distinct classes in the sense that diversity in the class is represented.
Following the formation of the clusters, ground truth validation is performed to determine the class to which the picture pixel belongs.
As a result, a priori information about the classes is not required in this unsupervised categorization. K-means clustering is a popular unsupervised classification approach.
Differences between Multispectral and hyperspectral
They are as follows:
Wavebands available
Multispectral imaging is performed using a Low Earth Orbit and is sun-synchronous. Data is collected by multispectral satellites over 5 to 10 bands of the spectrum. It frequently captures all three primary colors as well as a few blocks in the infrared section.
Hyperspectral imaging can identify thousands of different light spectrum bands.
If an analyst is aware with the spectral features of specific substances and minerals, such images can be incredibly useful in detecting them. Their satellites, like multispectral, follow the sun-synchronization, Low Earth Orbit.
Spectral resolution specifics
The number and width of electromagnetic spectrum components sensed by a sensor are referred to as spectral resolution.
The spectral resolution of multispectral remote sensing is weak. As a result, it is more difficult to distinguish the Earth’s features than hyperspectral sensors. Because of the vast bands, multispectral sensors are collected in tiny numbers.
Hyperspectral remote sensing, on the other hand, has a high spectral resolution that allows it to identify the spectral features of objects and minerals. It improves the ability to see the invisible.
The band’s narrowness
Parallel sensor arrays collect radiation in a small number of larger wavebands in multispectral remote sensing devices.
Meanwhile, the bands of hyperspectral remote sensing are substantially thinner. In hyperspectral sensors, the multiple small bands offer a continuous spectral measurement over the whole electromagnetic spectrum. As a result, they are more sensitive to minor fluctuations in reflected energy.
Knowledge of artificial intelligence and machine learning
Because multispectral remote sensing photos have a reduced informational quality, the same technique is used over and over again.
This imaging technology has a development handicap due to its lack of information richness.
Hyperspectral imaging technology, on the other hand, is aided by the high informative content, resulting in constant improvement. As a result, it is poised to become a main remote sensing technology used on a global basis.
Level of complexity
Data analysis and interpretation are simple in multispectral remote sensing due to the small number of bands. It is also simpler to comprehend.
Meanwhile, the complexity of hyperspectral remote sensing is one of its drawbacks. Because there are so many bands to deal with, it may be difficult to remove redundancies or hard work.
Features of the image
Reflected energy in the spectrum spans a broader range with multispectral data. It makes capturing a lot of detail on an object or surface area challenging. It is due to the fact that the wavelength bands are substantially wider.
When it comes to hyperspectral data, photos contain hundreds of points for each band, allowing for far more information to be observed.
The wavelength is divided into many tiny bands, each of which captures a unique spectral fingerprint or characteristic of an object.
As a result, the photos captured include significantly more data, prohibiting any analyst from detecting differences between land and ocean characteristics.
Camera distinctions
Multispectral images are taken using special cameras that use filters to separate wavelengths or equipment that are sensitive to specific wavelengths. These can include light from invisible to the human eye wavelengths.
Hyperspectral cameras, on the other hand, can detect a wide range of wavelengths independently. They can also see infrared and ultraviolet wavelengths, allowing them to see across a broader spectrum than humans.
As a result, in this imaging technique, analysts will obtain a 2-dimensional image in which each pixel has a continuous spectrum.
Costs
In most cases, multispectral sensors acquire data from three to six spectral bands in a single observation. These characteristics make them cost-effective. Because the image captures are simple, they are inexpensive to purchase and maintain.
Meanwhile, hyperspectral imaging allows for the collection of hundreds of spectral bands in a single acquisition. Such a feature has increased the cost because it necessitates greater technological innovation to obtain more detailed spectrum data.
This brings with it difficulties such as rising sensor and image expenses, data quantities and data-processing costs, and a high demand to keep operations running.
Pixel arrangement
Each pixel in multispectral remote sensing has a separate sample spectrum. Some wavebands, for example, may include 4 to 20 data points per pixel.
In contrast, each pixel in hyperspectral remote sensing has a continuous or entire spectrum.
Methods of processing
The multispectral remote sensors can only process a limited number of images.
Hyperspectral remote sensor processing approaches, on the other hand, include both spectral and picture data.
Advantages of Multispectral imaging
They include:
- Multispectral sensors are less costly, more easily acquired and have a high spatio-temporal resolution than hyperspectral sensors.
- Multispectral remote sensing is easy to use because it requires only a small number of samples.
- Multispectral remote sensing is highly effective in detecting static features, such as vegetation and water masses, and readily visible surface features, like crops or trees.
- High spatial resolution can be obtained using multispectral remote sensing.
- Remote sensing in the visible light spectrum can be achieved due to the narrow wavelength bands of multispectral remote sensing.
- Multispectral remote sensing is ideal for monitoring several fixed points over a long period of time because it is affordable and removes the need for daily measurements.
Disadvantages of Multispectral imaging
They include:
- The number of bands present in multispectral remote sensing is less than that which is available for hyperspectral imaging, making it difficult to achieve more detail.
- Multispectral remote sensing does not capture the whole spectrum simultaneously, unlike hyperspectral remote sensing.
- Its pixel resolution can be poor if there is a wide separator between adjacent bands.
- Multispectral imaging is not perfect in detecting minerals or sensitive materials that are invisible to the human eye.
- Multispectral remote sensing is incapable of detecting ultraviolet rays, which make up more than 97% of the light in the universe.
- The amount of information provided by a multispectral remote sensing can only be determined by the number of points captured at each wavelength interval. Even if these were increased, there would be a large degree of overlap between them.
Advantages of hyperspectral imaging
They are:
- Because hyperspectral remote sensing uses greater numbers of wavelengths, it is capable of capturing a large amount of information in a single photo-taking session.
- The photos captured by this imaging technique are far more detailed and accurate than those obtained using multispectral sensors as well as electromagnetic radiation such as radar and sonar.
- The image taken from an electromagnetic sensor contains highly detailed information about the volume, mass and energy contained within a certain area or object.
- Hyperspectral remote sensing has the capability to reveal subtle differences between the surface of land and water.
- Images taken using hyperspectral remote sensing are capable of detecting not only visible wavelengths but also invisible wavelengths such as infrared, ultraviolet and thermal.
- Hyperspectral remote sensing collects information on materials that are hidden or shielded from electromagnetic radiation such as radar, sonar and microwaves.
Disadvantages of hyperspectral imaging
They are:
- The effectiveness of hyperspectral imaging is limited by the fact that it uses a very large number of bands.
- Because hyperspectral imaging has to be taken from very low altitudes, it is difficult to acquire in some instances because clouds obscure the target images.
- Some targets are extremely hard to detect because they receive little energy from the sun, so they may be better suited for multispectral remote sensing.
- Hyperspectral imaging is limited to seeing light in the infrared and ultraviolet spectrum.
- Hyperspectral remote sensing cannot be used in multispectral remote sensing because it does not have the spatial resolution to sense a specific target or scene.
- The accuracy of hyperspectral imaging is poor at detecting some minerals, such as quartz and feldspar, because they are transparent to wavelengths below 0.75 micron.
FAQs
What is multispectral imaging?
Multispectral imaging is a remote sensing technology that captures light in the visible, near-infrared and short-wave infrared wavelengths.
What is hyperspectral imaging?
Hyperspectral imaging captures light in the visible, near-infrared and short-wave infrared wavelengths. It also captures other wavebands such as thermal infrared and ultraviolet spectra.
What is hyperspectral remote sensing?
In hyperspectral remote sensing, the data sample is a single image taken by a space borne platform or aircraft. However, in hyperspectral imaging, it refers to a specific spectral band that takes pictures in other areas of the spectrum such as thermal infrared and ultraviolet.
What is multispectral imaging used for?
Multispectral imaging detects and tracks military targets by measuring light emission.
How do multispectral cameras work?
Multispectral cameras capture images of various wavelengths of light. The camera’s output is a series of photos for that specific wavelength. These image sets are then stitched together to provide geographically accurate mosaics with many layers for each wavelength.
What is multispectral image in remote sensing?
Multispectral remote sensing entails acquiring images in visible, near infrared, and short-wave infrared wavelength ranges. At different wavelengths, different materials reflect and absorb differently.
What are multispectral sensors?
Sensors that measure reflected energy within many specific parts (also known as bands) of the electromagnetic spectrum generate multispectral images. Each pixel of an image produced by a multispectral sensor typically has between 3 and 10 distinct band measurements.
What is the difference between hyperspectral and multispectral images?
The fundamental distinction between multispectral and hyperspectral imaging is the number of bands and the narrowness of the bands.
Multispectral photography typically consists of three to ten bands. Hyperspectral imagery has significantly thinner bands (10-20 nm). There could be hundreds or thousands of bands in a hyperspectral photograph.
How do you get hyperspectral images?
Many different electromagnetic measurements can be used to generate hyperspectral images. The most common are visible (VIS), near-infrared (NIR), middle infrared (MIR), and Raman spectroscopy.
What is a limitation of hyperspectral images?
The biggest disadvantages are expense and complexity. To analyze hyperspectral data, fast computers, sensitive detectors, and vast data storage capacity are required.
Because hyperspectral cubes are big, multidimensional datasets that are likely to reach hundreds of megabytes in size, significant data storage capacity is required.
Who uses hyperspectral imaging?
In the food industry, hyperspectral imaging is commonly employed. It is employed in a variety of food industry disciplines, including apple bruise detection, fish freshness inspection, citrus fruit inspection, sugar distribution in melons, and potato sorting.
What is hyperspectral image classification?
The classification of hyperspectral images (HSI) is a fantastic approach for analyzing diverse land cover in remotely sensed hyperspectral pictures.
The main goal of hyperspectral image classification is to assign a class label to each pixel given a sequence of observations with known class labels.
How many bands are there in hyperspectral?
Hyperspectral data sets often consist of 100 to 200 spectral bands with relatively narrow bandwidths (5-10 nm), whereas multispectral data sets typically consist of 5 to 10 bands with relatively high bandwidths (70-400 nm).